Advanced Insight Module
The teams that keep IT services running all share one thing: a need for data and knowledge that spans their systems and tools. Yet they often lack the vital cross-system context necessary to analyze and collaborate effectively to remediate incidents quickly.
BigPanda’s Advanced Insight Module takes the overwhelming volume of context-rich incident data and surfaces actionable, plain-language insights for your team. Using generative AI and rich historical insights, the Advanced Insight Module can confidently and precisely determine incident impact. Use data from your entire IT stack to generate enriched context summaries that describe priority, scope, and assignment teams.
Leverage AI-powered insights backed by clear reasoning across your IT environment to deliver unified, full-context incident data to operations and ITSM teams to expedite triage and reduce MTTR. Get the complete picture every time to investigate and respond quickly and consistently, keeping services online.
Data Protection
Automated Incident Analysis leverages the OpenAI LLM, but the security of your data is our top priority. BigPanda sources its Generative AI language models using a version that protects the data shared externally. To learn more about how BigPanda protects your data, see our Trust Center.
BigPanda University Advanced Insight Module course
Learn more in the BigPanda University Advanced Insight Module course.
By the end of the course you will be able to:
Generate and review AI Analysis reports for your incidents that will help you reduce MTTR.
Review root cause change suspects and identify changes that are the root cause of incidents.
Compare incidents with similar characteristics to enhance context and resolve incidents faster.
Click here to enroll.
Key Features
Automated Incident Analysis
Root Cause Change Prediction
Similar Incidents
Automated Incident Analysis
BigPanda's Automated Incident Analysis leverages large language model AI to provide plain-language incident titles, detailed summaries, probable root cause analyses, and recommended actions built from enriched, actionable incident data.
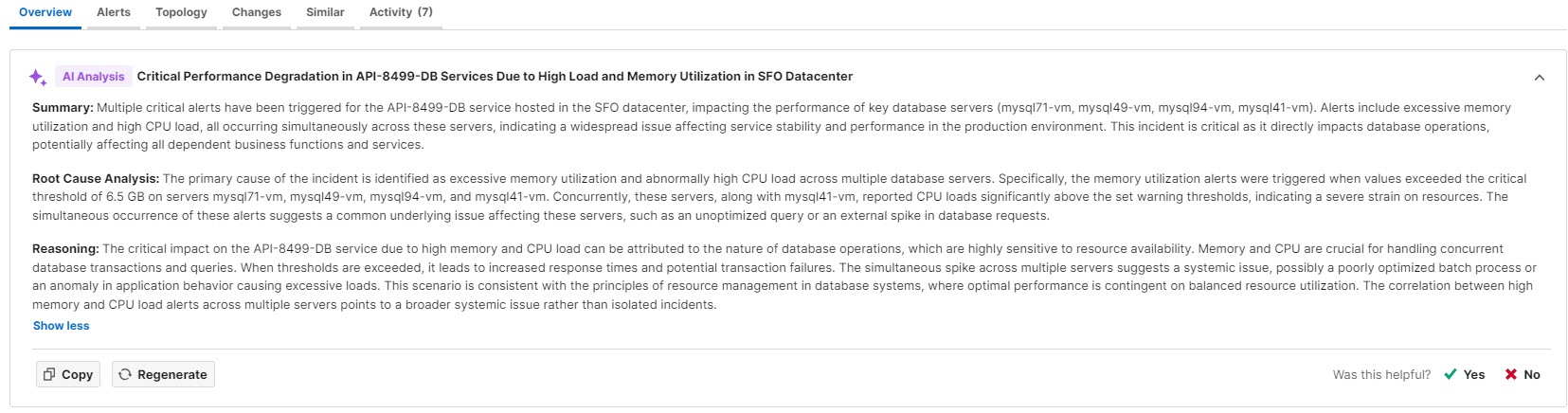
In environments where Automated Incident Analysis has been enabled, the AI generated title, summary, probable root cause analysis, and recommended actions will appear in the Incident Details pane after the initial delay. In environments where it has not been enabled, you can manually generate an incident summary by clicking the Click to perform AI Incident Analysis button.
Recommended actions
You can select which incident tags to base the recommended actions on. For more information, see the Recommended Incident Actions documentation.
You can also configure Automated Incident Analysis to automatically share incident summaries with other teams and platforms.
Learn more about how to speed up incident triage with automatic analysis in the Automated Incident Analysis documentation.
Root Cause Change Prediction
A significant portion of incidents and outages are caused by software and infrastructure changes. Identifying these changes quickly and accurately is a core goal and challenge of incident management.
Root Cause Changes (RCC) aggregates change data from all your change feeds and tools, including CI/CD, Change Management and Auditing. This complex data is then normalized, ensuring consistent information across the platform.
With this standardized, aggregated data, BigPanda uses an algorithm based on natural language processing and vector space models to compare changes to active incidents. Changes that are identified as potential root causes are surfaced right on the active incident where teams are working. AI-generated analysis of suspected root causes provides more context and better insights into matches, saving you even more time as you hunt down the root cause of an incident.
RCC can rapidly accelerate the root cause investigation process by identifying potential causes right at incident detection.
Learn more about marking suspect changes as matches in the Remediate Incidents documentation, or dig into the logic that drives successful root cause changes in the Root Cause Changes (RCC) documentation.
Similar Incidents
Similar Incidents turn historical knowledge into actionable, AI-driven data to manage active incidents.
Reduce manual investigation by surfacing similar incident context to deliver actionable insights. Similar Incidents compares live incident alerts with relevant operational data to expedite investigation, triage, and resolution. Use knowledge gained handling previous issues to get ahead of P0s and take action faster.
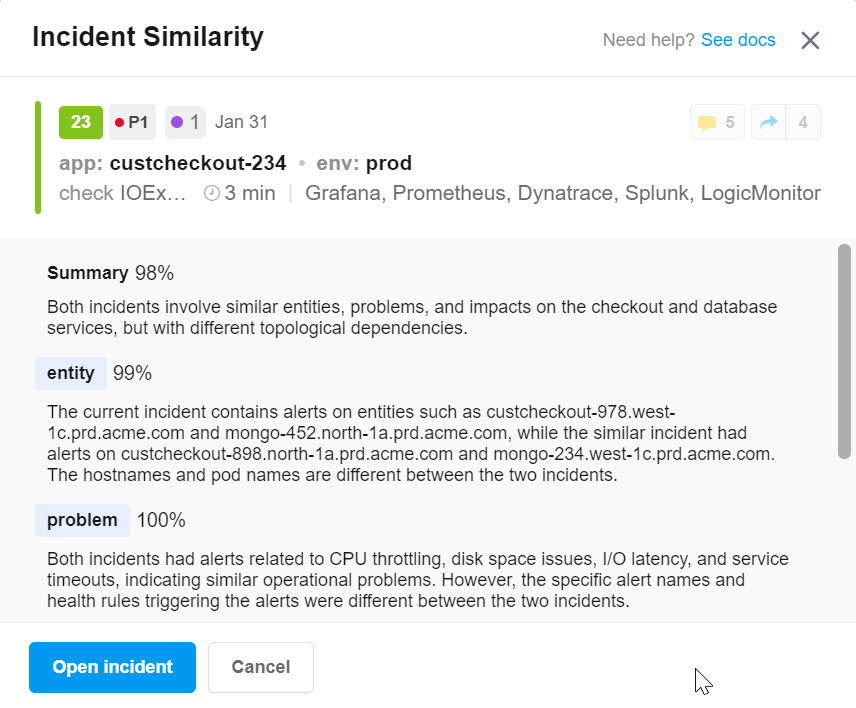
Similar incidents are chosen based on a similarity score. A list of the incidents most similar to the selected incident is generated each time you access the Similar tab within the Incident Details Pane. The score is calculated using similarity categories based on entity, problem, impact, and topology.
Learn more in the Similar Incidents documentation.
Next Steps
Learn more about how to accelerate incident investigation using Automated Incident Analysis.
Identify and review system changes that could be at the root of incidents using Root Cause Changes.
Reference incidents with similar characteristics to enhance context and resolve incidents faster using Similar Incidents.