Incident Intelligence
BigPanda’s Incident Intelligence service provides a powerful AI/ML-driven alert correlation engine that can help identify incidents in real-time, accelerate triage by adding business context and business logic, and surface probable root cause. Incident Intelligence enables faster incident detection, accelerated triage, and reduced escalations.
BigPanda correlates high-quality alerts together using AI/ML to identify high-level issues within your infrastructure, called incidents. By combining alerts from across your integrated monitoring systems, you gain visibility into the entire outage, helping you prevent these incidents from escalating into crippling outages.
BigPanda then adds operational and business context to incidents, including topology and service data, recent changes, relevant runbooks, and more.
This enrichment puts key knowledge at the fingertips of your IT Ops teams so that they can quickly sort, filter, visualize, and act on incidents. L1 operators have the knowledge to act and potentially resolve incidents without escalation, and SMEs no longer need to dig through external resources to understand the developing situation. With Incident Intelligence, you’ll need fewer bridge calls and manual coordination of effort, boosting the efficiency of IT Ops teams, and reducing MTTR.
![]() |
Algorithmic Correlation Process
BigPanda's Incident Intelligence provides:
Improved detection - Find critical issues faster as reduced noise surfaces important alerts before they become major outages.
Faster remediation - Get the full context of an incident in one place.
Better productivity - Reduce the number of tickets that operators have to handle, improving their ability to effectively manage emergency situations.
More control - Customize correlation patterns, enrichment tags, incident classification, and more to improve accuracy and efficiency.
Key Features
Alert Correlation
Incident Enrichment
Topology Integrations
Change Integrations
Incident Classification
Incident Console / API
Probable Root Cause
Alert Correlation
Correlation is the process of grouping related alerts into meaningful, actionable incidents. BigPanda uses pattern recognition to automatically process the data generated by your monitoring systems, dynamically clustering alerts based on the rich context available from the alert enrichment process. BigPanda provides default correlation patterns and gives you the option to tailor patterns to your organization.
BigPanda alert correlation engine clusters high-quality alerts into actionable incidents by looking at 4 properties:
Source System
Tags
Time Window
Filter (optional)
As new alerts are received, BigPanda evaluates all matching patterns and determines whether to update an existing incident or create a new incident. With this powerful algorithm, BigPanda can effectively and accurately correlate alerts to reduce your monitoring noise by as much as 90 – 99%. Correlations occur in under 100ms so you see updates in real time.
Correlation Patterns
Correlation patterns are high-level definitions that determine how alerts are clustered into BigPanda incidents. To increase the effectiveness of your alert correlation, you can customize the correlation pattern definitions in BigPanda based on the structure and processes of your company's production infrastructure.
For example, you can create patterns that correlate:
Network-related connectivity issues within the same data center.
Application-specific checks on the same host.
Load-related alerts from multiple servers in the same database cluster.
Low memory alerts on a distributed cache.
To learn more about defining and managing correlation patterns, see our Manage Alert Correlation guide.
Incident Enrichment
BigPanda takes the raw data from your systems and normalizes it into key-value pairs, called tags. Each tag has two parts: the tag name and the tag value. Tags are the fundamental data model for your alerts and incidents and provide vital incident enrichment.
In BigPanda, tags enable alert correlation, provide incident information in the UI, and help you configure environments, perform searches, and collect analytics.
Incident tags are a vital part of the incident management process, adding context and details to your BigPanda incidents, enabling better automation and faster troubleshooting. Incident tags are driven by both automatic incident enrichment formulas, and manual updates from team members through the UI.
Users are able to manually assign or remove incident tags. To learn more about using incident tags with incidents, please see the Add Tag Values to Incidents and Prioritize Incidents documentation.
You are able to create, edit, or inactivate incident tags to fit the needs of your organization. To learn more about configuring incident tags, please see the Manage Incident Enrichment documentation.
Incident tags may also be configured to automatically add to specific incidents based on incident or alert criteria. To learn more about configuring automatic tags, please see the Automatic Incident Tags documentation.
Topology Integrations
Using a growing number of standard integrations, BigPanda collects topology data from your common topology feeds and tools, and brings that data into a single location. The BigPanda Real-time Topology Mesh allows you to enrich, correlate, and route alerts based on topology data. In addition, the feature provides a visual representation of relationships and dependencies between the incident’s alerts.
BigPanda can connect to external topology sources via API or standard integrations. These sources are used to automatically enrich incoming alerts in real time. This drives several use cases:
Impact Assessment - Assess which business services and applications are impacted by low-level infrastructure issues.
Root Cause Analysis - Identify the common denominator or upstream potential causes of outages.
Incident Filtering & Routing - Easily isolate incidents based on their location in the infrastructure topology.
Correlation - Correlate events based on dependencies between networks, servers, clouds, and applications.
The Real-Time Topology Mesh feature relies on a combination of topology integrations, mapping enrichment, and topology visualization:
Enrich your alerts with a full-stack, always up-to-date topology model with BigPanda's topology integrations.
Digest topology and other enrichment information in real time and at scale with BigPanda's Mapping Enrichment API.
Visualize complex topology environments with automatically-generated graphs in the Topology tab of the incident details pane.
Change Integrations
Powered by a broad range of standard integrations for common change feeds, BigPanda can connect your tools together and aggregate that data in a single location. This data is normalized and organized into the Changes table in the incident details, where you can view managed changes, code deployments, software updates, and more while investigating, triaging, and remediating incidents.
To further automate and assist in troubleshooting change-related incidents, BigPanda’s Root Cause Changes (RCC) feature then correlates potentially related changes to incidents, helping you find and fix change-related incidents faster.
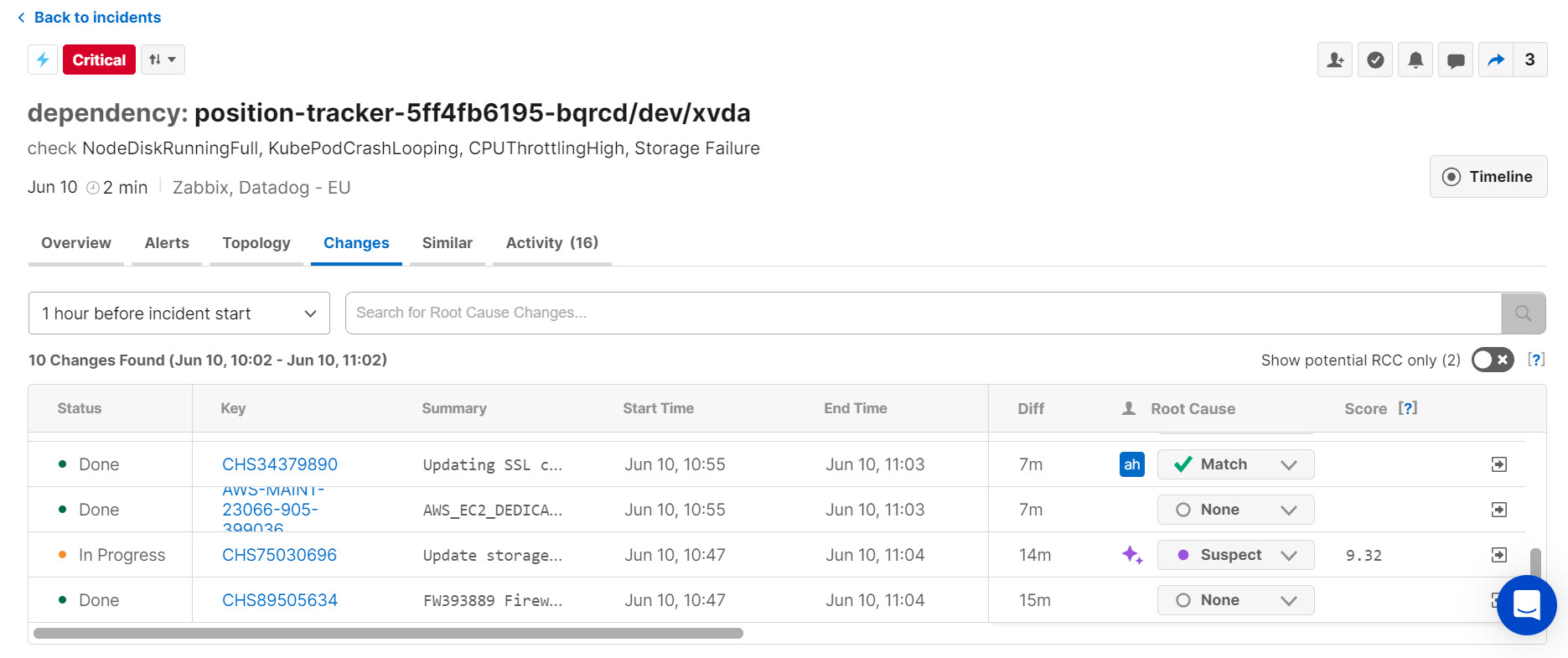
The Changes Tab
Read more about leveraging change data in the Remediate Incidents and Root Cause Changes documentation.
Incident Classification
IT Ops and NOC teams are often organized by defined roles, responsibilities, and access control levels across different shifts, geographies, and time zones. Similarly, different groups of incidents may have different sets of actions that need to be triggered, or shared to specific external teams, etc. A single ‘catch-all’ group of incidents for the entire organization is a barrier to ensuring RBAC or for triggering grouping-specific actions.
BigPanda’s environments filter and group incidents on properties such as source and priority for easy visibility and action. Environments make it easy for your team to focus on the incidents relevant to their role and responsibilities.
In addition to filtering the incident feed, environments are used to create analytics dashboards, set up sharing rules, and simplify incident search.
Learn more about using environments to view incidents in the Triage Incidents documentation, or start creating custom environments with the Manage Environments documentation.
Next Steps
With Incident Intelligence, your teams have all the knowledge they need to triage and remediate incidents faster. As your team focuses on actionable, high-context incidents, they’re able to triage and remediate issues before they become crippling outages.
To further speed up MTTR, BigPanda has tools to automate many of the initial manual actions that bog down ITOps teams responsiveness.
Read more about how BigPanda can help automate actions on your actionable incidents in the Workflow Automation documentation.
Or, if you’re ready to start customizing your Incident Intelligence process, check out the Manage Alert Correlation or Manage Incident Enrichment documentation.